Our demo videos showcase MaskHand's ability to reconstruct highly accurate and realistic 3D hand meshes from single RGB images, overcoming challenges like complex articulations, self-occlusions, and depth ambiguities.
MaskHand Training
MaskHand Training Phase . MaskHand consists of two key components: (1) VQ-MANO, which encodes 3D hand poses into a sequence of discrete tokens within a latent space, and (2) a Context-Guided Masked Transformer that models the probabilistic distributions of these tokens, conditioned on the input image, 2D pose cues, and a partially masked token sequence.
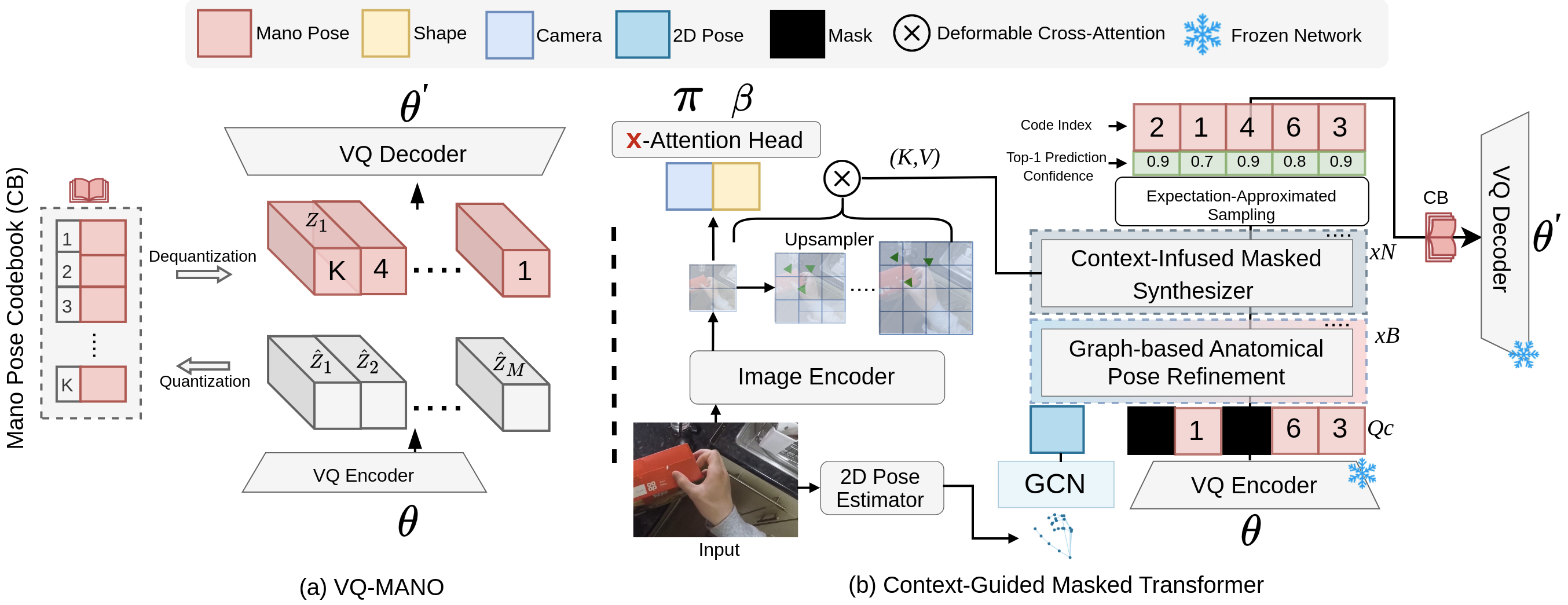
Text-to-Mesh Generation
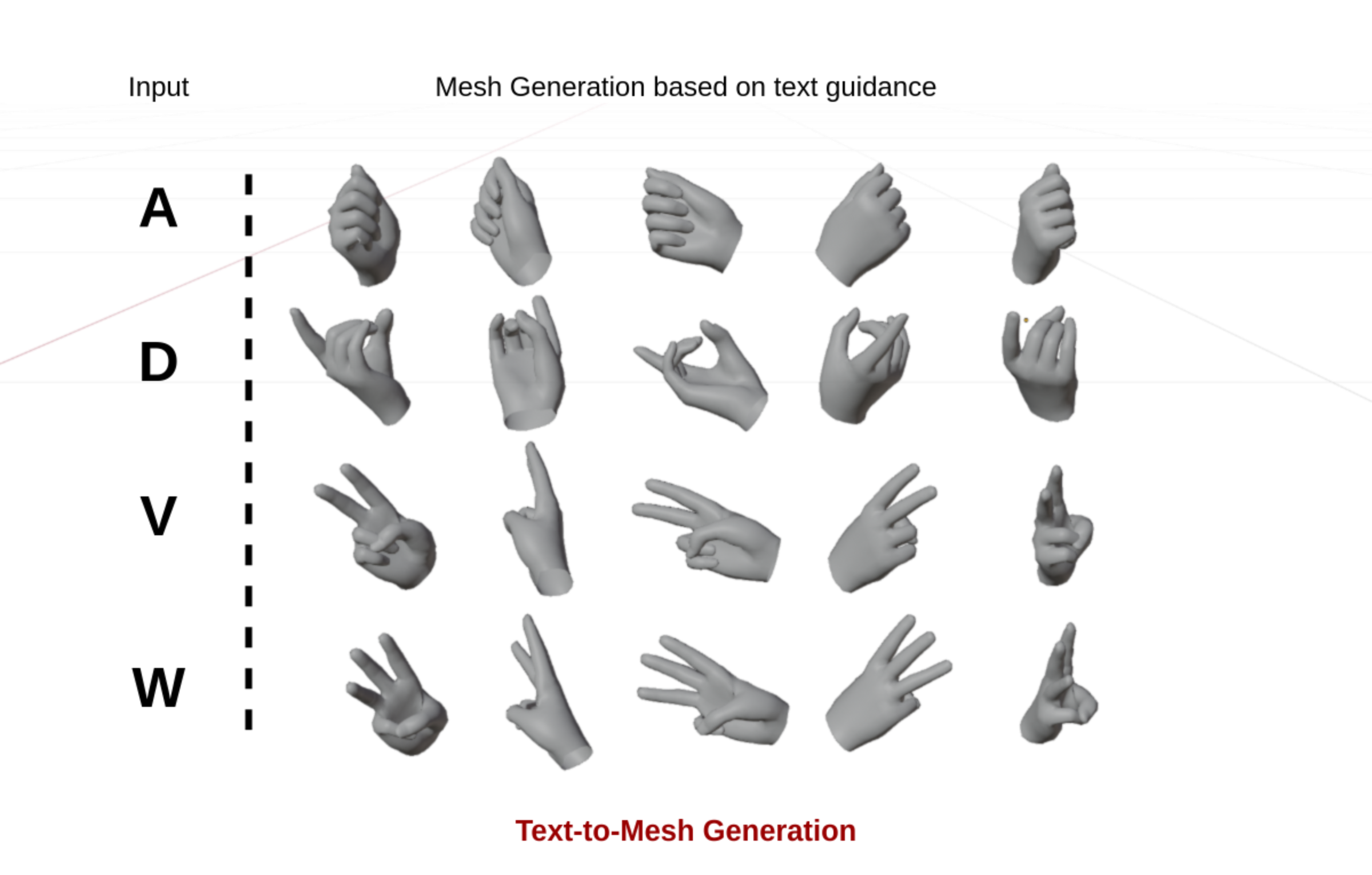
Confidence-Aware Unconditional Mesh Generation
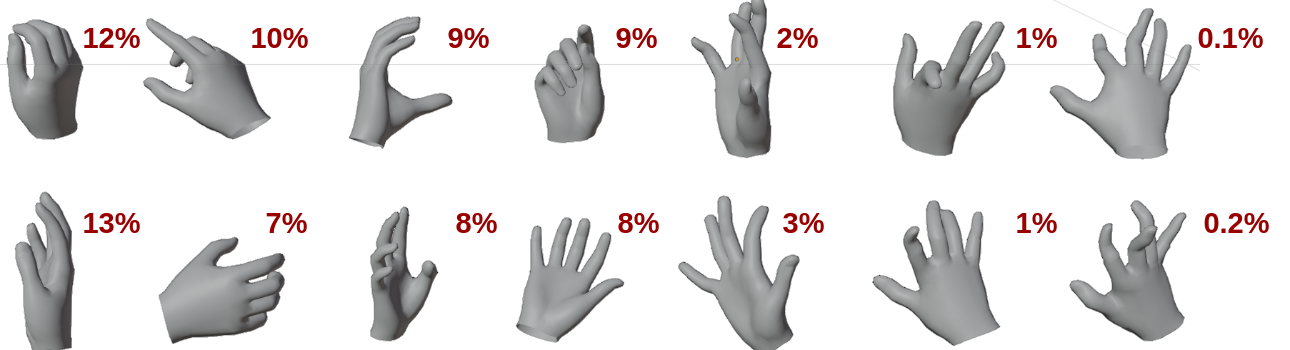